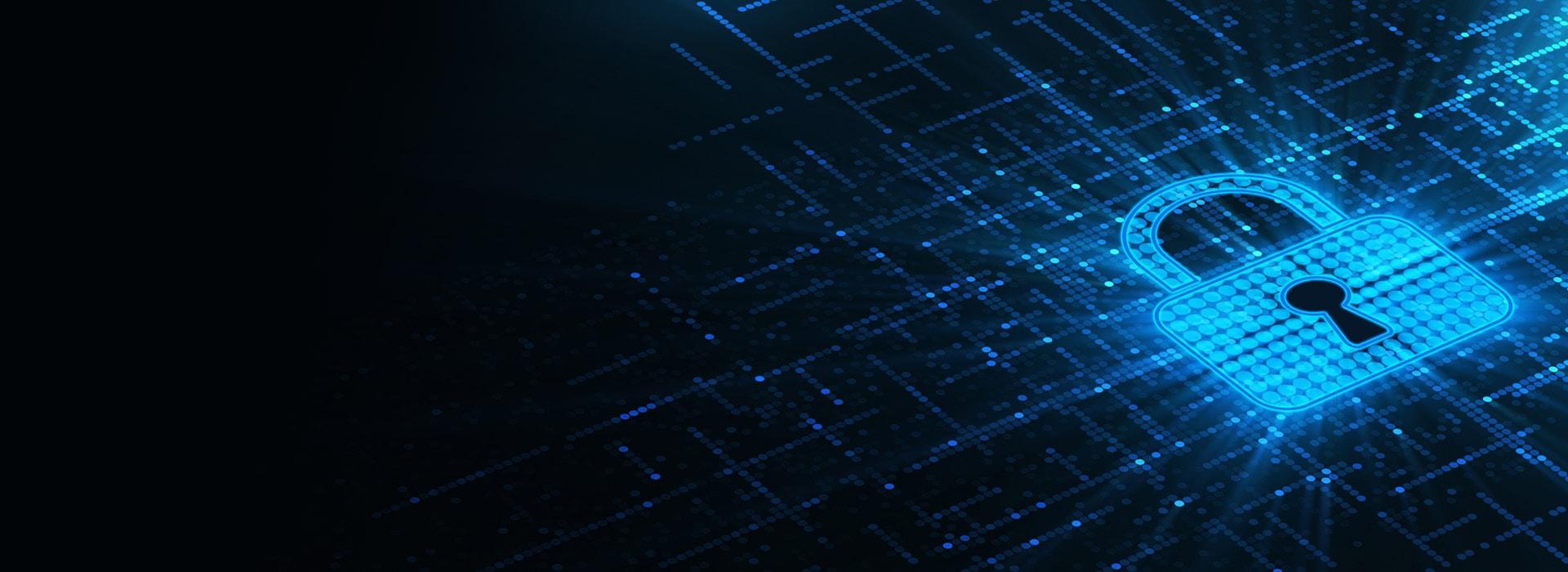
The term Big Data originated from the sheer amount of data generated every day in the era of computers. Though invaluable, this data deluge poses a formidable challenge: turning massive datasets into meaningful insights.Â
Then artificial intelligence (AI) solutions come into play. When it comes to resolving conflicts between big data and autonomous, well-informed direction, AI solutions are a remarkable force.Â
In this piece, we will examine the strong relationship between AI and big data and how these technological advancements work together to engage businesses of all kinds.
The Big Data Blast
A period of extraordinary data creation has been brought about by the development of the web, social media, IoT (Web of Things), and other cutting-edge technologies. Each web-based communication, sensor reading, and conversation produces a data point.Â
As businesses and people become progressively associated, the volume, velocity, and variety of data keep on developing dramatically.
The three facets of Big data—Volume, Velocity, and Variety—describe its enormous potential for growth and experiences.Â
Ultimately, the challenge is to extract meaningful patterns, noteworthy data, and meaningful patterns from this massive ocean of data. This is where the combined power of AI and big data becomes useful.
AI’s Part in Big Data Analytics
The main component in the field of Big Data analytics is AI reasoning, which includes machine learning, natural language processing, and advanced analytics. This is the way AI solutions overcome any barrier between the epic datasets and the choices that matter:
1: Data Entry and Research
AI succeeds in dealing with the gigantic volumes of data that Big Data entails. Machine learning algorithms are capable of analyzing and interpreting both structured and unstructured data. It reveals insights and identifies patterns that traditional scientific methods might miss.
2: Predictive Analytics
Utilizing verifiable data, AI models can make predictions about future patterns, ways of behaving, and results. This ability is invaluable for companies moving from finance and healthcare to marketing, as it empowers proactive dynamics in front of data rather than reactive responses.
3: Regular Language Processing (NLP)
Unstructured data, for example, text from social media, customer reviews, and articles, holds gigantic value. AI, especially NLP, can dissect and figure out human language, transforming unstructured text into structured data for additional investigation. This ability is essential for feeling investigation, customer criticism, and market patterns.
4: Personalization and Recommendation Systems
Big Data-filled AI-powered recommendation engines drive platforms like Netflix, Amazon, and Spotify. These systems look into customer behavior and preferences to provide personalized recommendations that enhance customer loyalty and experience.
5: Automation of Recurrent Activities
AI succeeds in automating complex and time-consuming errands. In terms of Big Data analytics, this means automating preprocessing, data cleaning, and report creation. This reduces the chance of human error associated with manual data entry and saves time.
How about going further into the particular ways AI overcomes any barrier between big data and big decision:
A. Design Acknowledgment and Forecast
Like a carefully prepared analyst, AI succeeds at spotting connections and irregularities in data. It can distinguish complex patterns that natural eyes could miss, anticipating future patterns with amazing precision. This enables businesses to remain on the ball, anticipating customer needs, enhancing inventory management, and anticipating market shifts before they hit.
B. Customized Experiences
Customizing experiences is essential in today’s customer-driven world. AI deconstructs individual data sets to determine preferences, past purchases, and online behavior. Subsequently, this data is utilized to personalize content, offers, and recommendations, encouraging increased client loyalty and commitment. Imagine an online store that makes surprisingly great recommendations for products based on your previous purchases.
C. Operational Proficiency and Risk Management
AI isn’t just about grasping customers; it also improves inward cycles. Artificial intelligence (AI) analyzes operational data to identify weaknesses, identify potential bottlenecks, and computerize tedious tasks.Â
This reduces expenses, streamlines operations, and frees up HR for higher-level work. AI can also analyze data to identify security threats, anticipate equipment failures, and mitigate risks before they materialize, strengthening and foreseeing an organization.
D. Data Democratization and Collaboration
Once, data analysts and researchers were confined to their ivory towers and handled only big data. AI solutions democratize data, making experiences open to all levels of the organization. Artificial intelligence (AI) makes complex data understandable to all through natural dashboards and visualizations, promoting cross-divisional collaboration and data-driven decision-making.
E. Ethics and Simpleness
No matter how powerful AI gets, it comes with ethical reflection. Data predisposition can lead to biased computations that maintain inequality and segregation. Dependable organizations should guarantee their AI solutions are easy, fair, and responsible. Explainable AI strategies are essential, allowing clients to comprehend how choices are made in light of data, building trust, and moderating possible moral worries.
Uses of AI and Big Data Integration
1. Healthcare: Accuracy Medicine
In healthcare, the integration of AI and Big Data is changing patient considerations through accurate medicine. Artificial intelligence (AI) algorithms can identify personalized treatment plans based on an individual’s genetic makeup and medical history by analyzing large datasets that include genomic data, patient records, and therapy outcomes. This approach improves treatment viability as well as limits possible incidental effects.
2. Finance: Fraud Detection and Risk Management
In the financial sector, fraud detection and risk management heavily rely on the combination of AI and Big Data. Machine learning calculations look into exchange trends, client conduct, and verifiable data to recognize inconsistencies that might demonstrate fraudulent exercises. This proactive methodology empowers monetary establishments to relieve chances and safeguard their customers.
3: Retail: Optimizing the customer experience
Retail monsters influence AI and Big Data to enhance the customer experience. Retailers can tailor showcasing campaigns, provide targeted advancements, and enhance inventory management by analyzing customer behavior, purchase history, and preferences. This upgrades customer fulfilment as well as works on operational effectiveness.
4. Manufacturing: Predictive Maintenance
In the manufacturing industry, AI and Big Data integration work with predictive maintenance. AI models can predict when equipment is likely to break by analyzing data from sensors inserted into machinery. This allows for proactive maintenance and the reduction of idle time. This predictive methodology lessens operational expenses and broadens the life expectancy of machinery.
Wrapping Up
Big data and big decisions are closely related, but the relationship between them is dynamic and ever-evolving. You can expect far more sophisticated solutions as AI technology develops, ones that can handle ever more complex datasets and yield much deeper experiences.Â
This offers organizations two opportunities as well as challenges. Data-driven navigation will benefit those who adopt AI and use it consistently, while those who fall behind run the risk of becoming obsolete in the rapidly evolving computer landscape.